How to Prepare Your Infrastructure for LLMs and Generative AI
We find ourselves amidst the era of Large Language Models (LLMs) and Generative AI, yet it’s crucial to pause and contemplate the implications for our technological infrastructure. While the necessity for more data and increased capacity is evident, have we considered the fundamental shifts LLMs and Generative AI will introduce to our traditional scaling methods for data infrastructure? Acknowledging the massive data requirements is just scratching the surface.
It’s essential to delve into how LLMs and Generative AI will redefine innovation. Understanding the transformative impact of these technologies on the data landscape is a starting point. From there, we can strategize on how to equip our infrastructure for the forthcoming digital ecosystem.
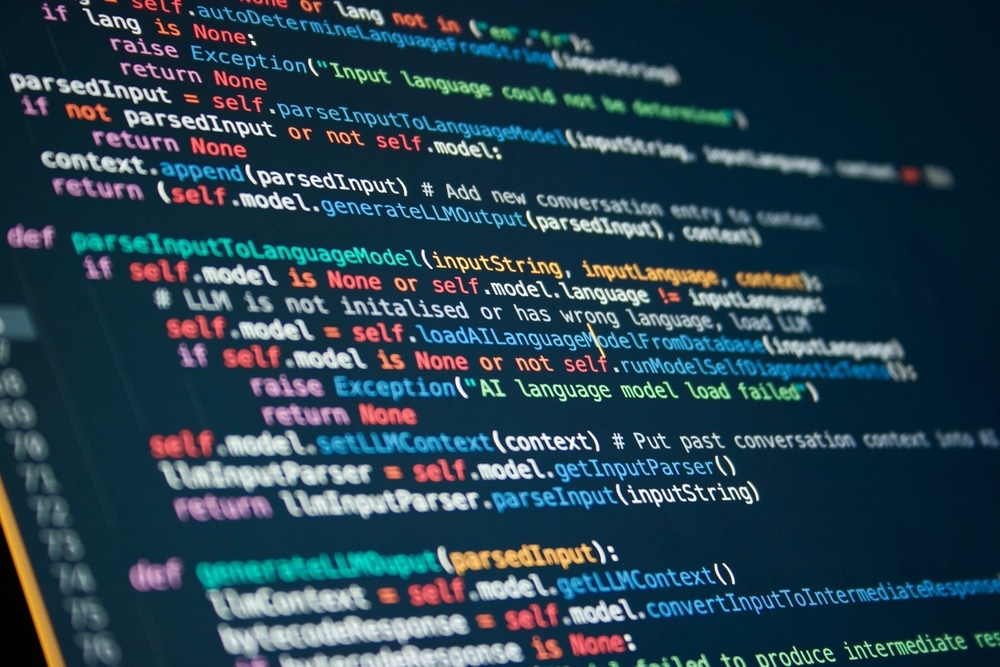
What are LLM (Large Language Models) and their Role in Modern Tech
Large Language Models (LLMs) represent AI systems engineered to decipher and replicate human speech patterns. With an LLM at its core, tools like ChatGPT can swiftly furnish responses and insights to fundamental queries. These systems can generate a 500-word essay almost instantaneously.
Training LLMs demands copious amounts of data, typically in text form. Rather than ‘thinking’ akin to humans, LLMs learn to predict human-like text generation. However, the scalability of this ability is remarkable. Tools such as OpenAI’s GPT-3, Stable Diffusion (for images), and even audio applications like OpenAI Whisper emulate a remarkably human-like experience. They offer customized audio and visual outputs based on simple prompts provided by humans.
The real question isn’t about the utility of LLMs. Their effectiveness is already evidenced by simplifying AI interactions, allowing users without specific skills to elicit professional-grade outcomes rapidly. The true query revolves around whether your computing infrastructure can handle the advantages of AI.
This isn’t a trivial consideration. Microsoft made headlines recently by flirting with the idea of using nuclear power its AI ambitions. This highlights the substantial computing power required by large-scale operations to effectively leverage LLMs and generative AI.
Understanding the “Generative AI Flywheel”
Generative AI utilizes the outputs of LLMs to produce what appears to be fresh, original content. For instance, asking ChatGPT for an essay on the history of France results in it instantly “generating” one. Similarly, instructing Stable Diffusion to create a specific type of image results in it promptly “generating” the requested image.
In this context, the concept of a Generative AI “flywheel” becomes increasingly critical for tech companies. Unfamiliar with the term? A flywheel is a self-reinforcing cycle where the momentum from preceding steps imparts energy to the subsequent ones.
For Generative AI, this flywheel signifies that each iteration becomes more potent as more data is accumulated and integrated into the machine learning model. More data equates to heightened accuracy.
Establishing a flywheel necessitates the collection and maintenance of vast quantities of data. For instance, developing an AI-powered self-driving car demands an array of data from car cameras to keep the vehicle aware of its surroundings. The larger the volume of data, the more extensive the sample size, ultimately enhancing AI accuracy.
As the AI grows in accuracy, its predictive capabilities improve. In the case of ChatGPT, refined “predictions” entail better anticipation of the subsequent words in each response. This culmination of more accurate predictions results in more precise outcomes, creating a scenario where the whole becomes greater than the sum of its parts. The AI appears almost uncanny in its ability to comprehend and respond to various prompts or scenarios.
This evolution bodes well for the future of AI: larger sample sizes enhance its intelligence over time. However, this also underscores the necessity for a robust infrastructure capable of supporting vast quantities of data.
Prepping Your Infrastructure for the LLM/Generative AI Future
Certainly, ensuring a robust infrastructure for the era of AI, particularly when working with Language Learning Models (LLMs) and Generative AI, requires more than just massive data storage. Delving into the specifics, let’s outline key elements that constitute a supportive infrastructure:
- High-Performance Computing: The strength of your hardware is crucial. GPUs adept at handling Generative AI for imaging and video processing are vital for many companies. Moreover, having CPUs optimized for AI workloads is essential. Raw processing power is indispensable to effectively manage the level of AI performance you aim to achieve.
- Scalable cloud infrastructure: Cloud services like AWS, Azure, or Google Cloud can make your cloud infrastructure’s pricing easier to predict and scale. With scalable cloud infrastructure, you can adjust your storage based on usage. You don’t have to worry about over-doing it on the storage side. You also don’t have to worry about playing catch-up when you need storage in a hurry. You might also consider cloud storage as a service, as this will create a scalable infrastructure with predictable pricing.
- Data Storage and Management: Creating a comprehensive plan for storing and safeguarding vast amounts of data is critical for initiating a Generative AI flywheel within your organization. With a flywheel, the objective is to build upon successful results. This necessitates effectively managing and retaining older data, potentially requiring future scalability in data storage solutions.
These strategies form a foundational framework for designing a data infrastructure suitable for AI’s demands. However, let’s outline specific steps to craft a dedicated and focused plan for developing your infrastructure:
- Evaluate your current situation. Building a map for the future requires a thorough evaluation of your current situation and what it’s capable of doing. Look at your data storage solutions. If you suddenly needed to store twice the data, how would you approach it? Which systems might you need to implement?
- Create data governance policies. With more data, you’ll have a greater need to govern, regulate, and secure that data. So as you begin planning for the future, draw up a list of policies to secure the data you collect. Establish good habits from the get-go so your team is used to them as you scale.
- Implement data catalogs with proper metadata labeling. Knowing your metadata will ensure that as you collect the large quantities of data you need for Generative AI and LLMs, you’ll need a way to catalog and label that data and make it easier to sift through.
Preparing your infrastructure for LLMs and Generative AI doesn’t have to be an intimidating process. Break it up into smaller pieces, set clear, definable goals along the journey, and prepare for a future where data is the key to the quality of your AI. Because that future is already here. For more information, contact our team.